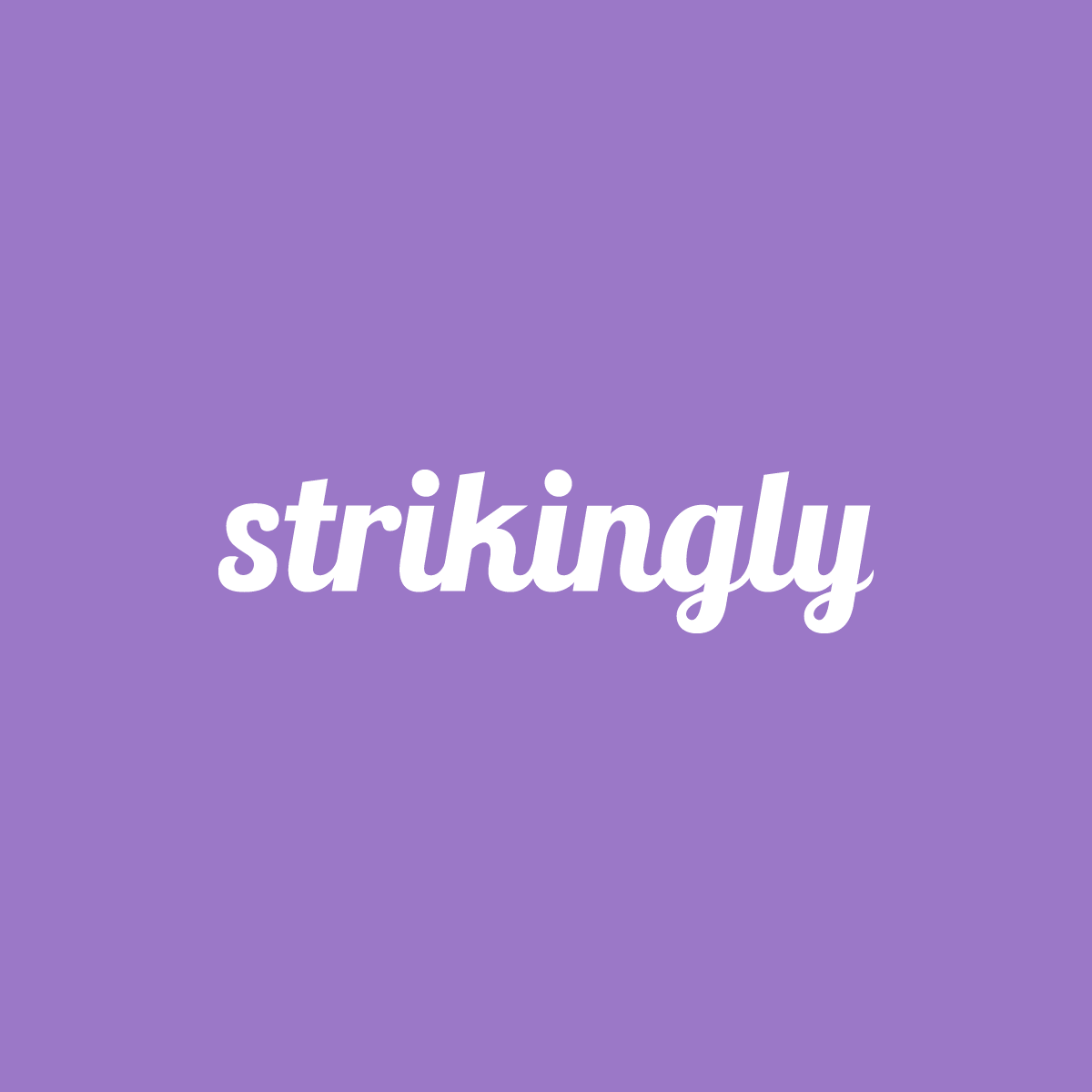
- Bhavin S Khatri
Understanding how evolution works using tools of statistical physics
- ResearchEvolution of Biophysical Genotype Phenotype maps
Using analogies from statistical physics to understand emergent phenomena in evolution
Natural selection acts on phenotypes, yet variation in phenotypes arises from mutations at the genetic level. It is being increasingly recognised that a crucial missing ingredient for a full understanding of evolution, even at a qualitative level, is the role of genotype-phenotype maps, or the mapping from sequence to function. Quantitative and predictive theories of evolution that account for this mapping will be required to understand several pressing problems, including antibiotic resistance, virus evolution, and cancer evolution. A key generic prediction is that small drift-dominated populations adapt to phenotypes that have the most sequences coding for them instead of the most optimal (Khatri, PNAS 2009, Genetics 2016). This means smaller populations undergo more rapid speciation as common ancestors are more likely to be maladapted (Khatri, JTB 2015, Khatri, Genetics, 2015 & Khatri, PLoS Computational Biology 2019). In addition, I have developed a stochastic theory for the evolution of phenotypes within a continuous stochastic dynamics framework (Khatri et al, JTB, 2015); this holds promise of greatly simplified modelling of complex genotype-phenotype maps in the future.
Virus EvolutionDetecting selection in longitudinal deep sequencing of viruses
My work aims to understand the basic evolutionary forces that shape the genomes of pathogens and the stochastic dynamics of variants in a host. A key quantity to calculate is the probability of observing a change in the frequency of a variant in a fixed time interval. This is a difficult mathematical diffusion problem that has eluded accurate solution for changes over short times. The key difficulty is the that the variance of allele frequencies (or diffusion constant) is not the same for all allele frequencies. Fisher's angular transformation gives a variance which is independent of a new angular frequency and so effectively simple Brownian motion. The cost is a non-linear potential which is a manifestation of the effects of genetic drift, and is probably why this transformation has largely gone unnoticed and unexploited, despite Fisher discovering it almost a century ago. To make practical use of the transformation, I developed an heuristic Gaussian-based method that gave simple and accurate solutions including genetic drift, selection and mutation. This work (Khatri, Sci. Rep. 2016) is a key foundational basis for analysing longitudinal deep-sequencing data. More recently, I used the same framework for a new analysis which can distinguish between transmission and intrahost fitness effects in phylogenetic trees (Khatri et al, in preparation) and a new method to calculate the rate of fixation of rare variants (Khatri, bioRxiv123232, 2017).
Publications
Weakly deleterious natural genetic variation greatly amplifies probability of resistance in multiplexed gene drive systems, Bhavin S. Khatri and Austin Burt, bioRxiv doi.org/10.1101/2021.12.23.473701
Stochastic extinction of epidemics: how long would it take for SARS-Cov-2 to die out without herd immunity? B. S. Khatri, medRxiv doi:10.1101/2020.08.10.20171454v3
From genotypes to organisms: State-of-the-art and perspectives of a cornerstone in evolutionary dynamics, Susanna Manrubia, José A. Cuesta, Jacobo Aguirre, Sebastian E. Ahnert, Lee Altenberg, Alejandro V. Cano, Pablo Catalán, Ramon Diaz-Uriarte, Santiago F. Elena, Juan Antonio García-Martín, Paulien Hogeweg, Bhavin S. Khatri, Joachim Krug, Ard A. Louis, Nora S. Martin, Joshua L. Payne, Matthew J. Tarnowski, Marcel Weiß, Physics of Life Reviews, 38, Pages 55-106 (2021)
Computational and experimental analysis of mitotic chromosome formation pathways in fission yeast, Tereza Gerguri, Xiao Fu, Yasutaka Kakui, Bhavin S. Khatri, Christopher Barrington, Paul A. Bates, and Frank Uhlmann, Nucleic Acids Research, 9, p1294-1312, (2021)
Fission yeast condensin maintains interphase chromatin organization to prevent transcription-coupled DNA damage
Yasutaka Kakui; Christopher Barrington; David J Barry; Tereza Gerguri; Xiao Fu; Paul A Bates; Bhavin S Khatri, Frank Uhlmann. Genome Biology, 21:272 (2020).Biophysics and population size constrains speciation in an evolutionary model of developmental system drift, B.S. Khatri and R. A. Goldstein, PLoS Computational Biology, 15(7): e1007177, https://journals.plos.org/ploscompbiol/article?id=10.1371/journal.pcbi.1007177, 2019
Robust estimation of recent effective population size from number of independent origins in soft sweeps, B.S. Khatri & A. Burt, Molecular Biology and Evolution, msz081, doi:10.1093/molbev/msz081 2019
Survival of the frequent at finite population size and mutation rate, B. S. Khatri, bioRxiv doi: https://doi.org/10.1101/375147 2018
Rate of fixation of rare variants in a population, B. S. Khatri, bioRxiv doi:10.1101/123232 2017
Optimal chemotactic responses in stochastic environments, M. Godany, B. S. Khatri and R.A. Goldstein, PLoS ONE, 12(6):e0179111, 2017
Quantifying evolutionary dynamics from variant-frequency time series, B. S. Khatri, Scientific Reports, 6:32497, 2016.
Simple Biophysical Model Predicts Faster Accumulation of Hybrid Incompatibilities in Small Populations Under Stabilizing Selection, B. S. Khatri and Richard A. Goldstein, Genetics, 201(4), 1525-1537, 2015.
Multiple-line inference of selection on quantitative traits, N. Riedel, B. S. Khatri, M. Lässig and J. Berg, Genetics, 201(1), 305-322, 2015.
A coarse-grained biophysical model of sequence evolution and the population size dependence of the speciation rate, B. S. Khatri and R. Goldstein, Journal of Theoretical Biology, 378, 56-64, 2015.
Oscillating microbial dynamics driven by small populations, limited nutrient supply and high death rates, B. S. Khatri, A. Free and R. Allen, Journal of Theoretical Biology, 314, 120{129, 2012.
Fluctuation power spectra reveal dynamical heterogeneity of peptides, B. S. Khatri, Z.T. Yew, T.C.B. McLeish, E. Paci, Journal of Chemical Physics, 133, 015101, 2010.
Dynamics of the coiled-coil unfolding transition of myosin rod probed by dissipation force spectrum, Y. Taniguchi, B. S. Khatri, D. J. Brockwell, E. Paci and M. Kawakami, Biophysical Journal, 99, 257-262, 2010.
Statistical Mechanics of Convergent Evolution in Spatial Patterning, B. S. Khatri, T.C.B. McLeish and R.P. Sear, PNAS, 106, 9564-9569, 2009.
Internal Friction of Polypeptide Chains at High Stretch, B. S. Khatri, K. Byrne, M. Kawakami, D.J. Brockwell, D. A. Smith, S.E. Radford and T.C.B. McLeish, Faraday Discuss., 139, 35 - 51, 2008.
Rouse Model with Internal Friction: A Coarse Grained Framework for Single Biopolymer Dynamics, B. S. Khatri and Tom C.B. McLeish, Macromolecules, 40 (18), 6770 -6777, 2007.
Entropy and Barrier-Controlled Fluctuations Determine Conformational Viscoelasticity in Single Biomolecules, B.S. Khatri, M. Kawakami, K. Byrne, D.A. Smith, T.C.B. McLeish, Biophysical Journal, 92, 1825-1835, 2007.
Viscoelastic Properties of Single Poly(ethylene glycol) Molecules, M. Kawakami, K. Byrne, B.S. Khatri, T.C.B. McLeish, and D.A. Smith, ChemPhysChem, 7, 1710 -1716, 2006.
Viscoelastic Measurements of Single Molecules on a Millisecond Time Scale by Magnetically Driven Oscillation of an Atomic Force Microscope Cantilever, M. Kawakami, K.Byrne, B.S. Khatri, T.C.B. McLeish, S.E. Radford and D.A. Smith, Langmuir, 21, 4765-4772, 2005.
Viscoelastic Properties of Single Polysaccharide Molecules Determined by Analysis of Thermally Driven Oscillations of an Atomic Force Microscope Cantilever, M. Kawakami, K. Byrne, B.S. Khatri, T.C.B. McLeish, S.E. Radford and D.A. Smith, Langmuir, 401, 400-403, 2004.
Contact
Email
bhavin.khatri@
physics.org Research Fellow in Statistical Genomics
Imperial College London
Department of Life Sciences
Silwood Park
&
Visiting Scientist
The Francis Crick Institute
London